Transform Your Business with Labelling Tool Machine Learning
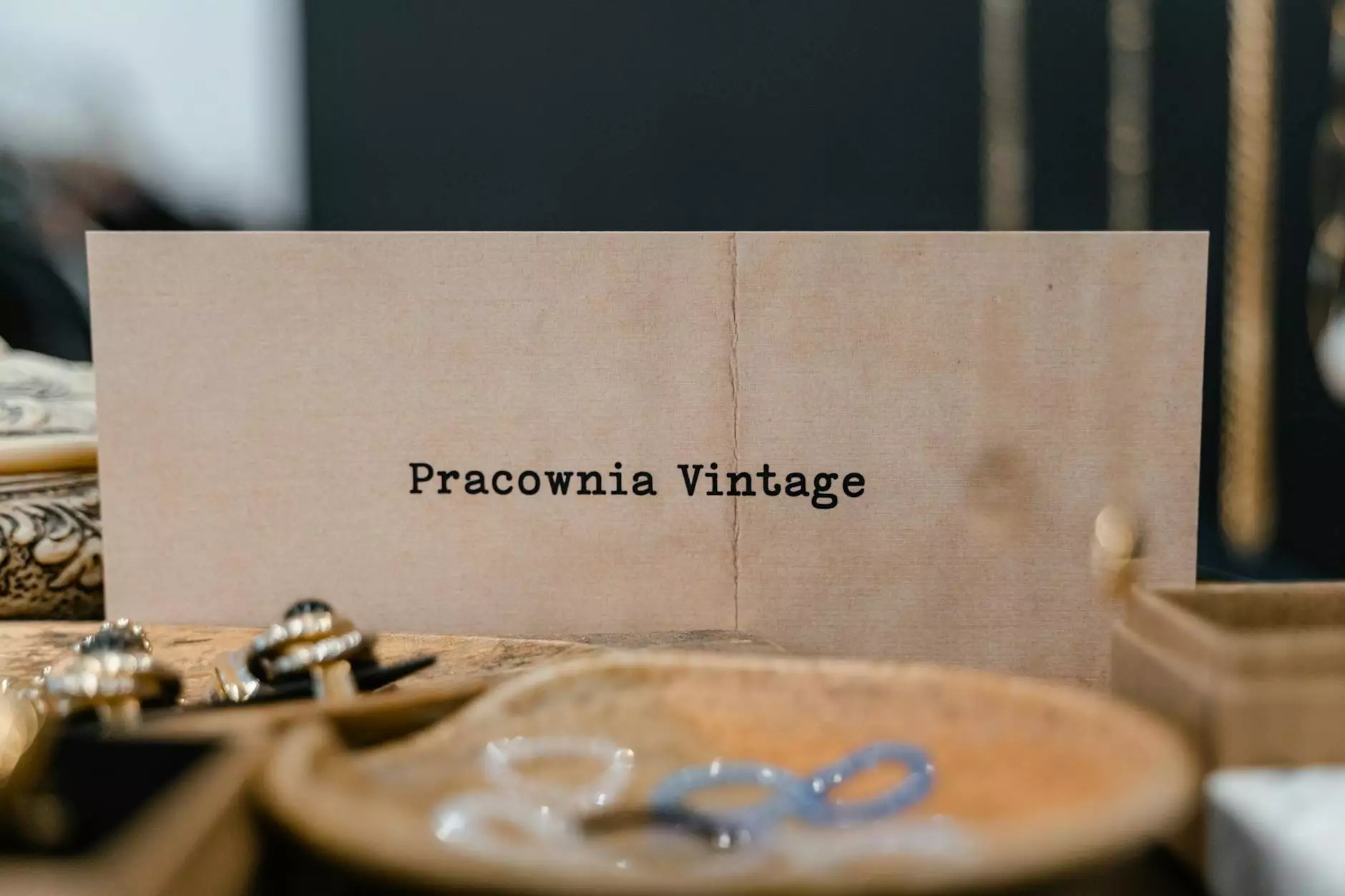
In an increasingly data-driven world, businesses are striving for an edge in making sense of vast amounts of information. One of the most transformative technologies in this domain is the labelling tool machine learning. By automating the data annotation process, these advanced tools significantly enhance operational efficiency, driving better decision-making and more accurate insights.
Understanding the Importance of Data Annotation
Data annotation is the process of labeling raw data to make it usable for machine learning models. It serves as the groundwork for training algorithms to recognize patterns, make predictions, and automate various tasks. Essentially, accurate and high-quality data annotations are crucial for the success of machine learning initiatives.
The Role of Labelling Tool Machine Learning
A labelling tool machine learning is designed to simplify and streamline the data annotation process, allowing for quicker turnaround times and higher accuracy. The integration of machine learning within these tools enables them to learn from existing data and improve over time, ensuring that the annotations provided are both consistent and precise.
Key Benefits of Using Labelling Tools in Machine Learning
- Increased Speed: Machine learning algorithms can handle large datasets faster than manual methods, drastically reducing the time required for labeling.
- Scalability: As your data needs grow, labelling tools can easily scale up, allowing businesses to manage increased data loads effectively.
- Enhanced Accuracy: With advanced algorithms, these tools produce highly accurate labels, minimizing human error.
- Cost-Effectiveness: Automated labelling reduces labor costs and reallocates resources to more strategic activities.
How Labelling Tool Machine Learning Works
The functionality of a labelling tool machine learning can generally be broken down into a few core processes:
1. Data Input
The first step involves the input of raw data into the labeling tool. This data can originate from a variety of sources, including text documents, images, audio files, and more. Depending on the tool, different formats may be accepted.
2. Pre-Processing
Once the data is inputted, pre-processing occurs. This step can include cleaning the data, standardizing formats, and preparing it for the machine learning algorithms that will be applied. Good pre-processing ensures that the data is in the best possible shape for annotation.
3. Machine Learning Labeling
The machine learning algorithms analyze the data, identifying patterns and making predictions on the most appropriate labels. Depending on the sophistication of the tool, it may offer various levels of human intervention to ensure that the final labels meet desired quality standards.
4. Human Review (if necessary)
In many cases, a human reviewer may be involved to validate the labels produced by the machine learning tool, especially in complex scenarios. This blend of automation and human insight often yields the best results.
5. Output Generation
The final output is generated, which consists of the fully annotated dataset. This annotated data can then be utilized in training machine learning models, leading to enhanced performance and accuracy in predictive analytics.
Choosing the Right Labelling Tool Machine Learning for Your Business
When selecting a labelling tool machine learning, consider the following factors:
- Functionality: Ensure the tool supports the types of data you will be working with and offers functionalities that meet your business needs.
- User Interface: The interface should be user-friendly, minimizing the learning curve for your team.
- Integration Capabilities: The tool should easily integrate with your existing systems and workflows.
- Cost: Evaluate the pricing structure to ensure it aligns with your budget while still providing the necessary features.
- Support and Training: Look for a provider that offers robust support services and training resources.
Case Studies: Successful Implementations of Labelling Tools
Many organizations have successfully leveraged labelling tool machine learning to enhance their operations. Here are a few examples:
1. E-Commerce: Product Image Tagging
One major e-commerce retailer utilized a labelling tool powered by machine learning to automate the tagging of product images. By significantly speeding up the process, they improved their inventory management and enhanced the shopping experience for their customers.
2. Healthcare: Medical Image Analysis
A healthcare company adopted a labelling tool to annotate medical images for training diagnostic algorithms. This resulted in faster diagnosis times and improved accuracy in identifying diseases, ultimately benefiting patient care.
The Future of Labelling and Machine Learning
As machine learning continues to evolve, so will the capabilities of labelling tools. Innovations such as neural networks, deep learning, and advanced natural language processing are on the horizon, promising to enhance the speed and accuracy of labelling even further.
Businesses that invest in these emerging technologies will gain a competitive edge, positioning themselves as leaders in their respective fields. The transition to automated labelling processes will not only streamline operations but also enable data-driven decision-making and strategic growth.
Conclusion: Embrace the Power of Labelling Tool Machine Learning
In conclusion, the adoption of a labelling tool machine learning is a game-changer for businesses looking to optimize their data annotation processes. By using these advanced tools, organizations can improve efficiency, reduce costs, and harness the full potential of their data. Embrace the future of intelligent data management, and watch as your business thrives in the digital era.
Take Action Now!
If you are ready to transform your data annotation process, explore the powerful offerings at keylabs.ai. Experience firsthand how labelling tool machine learning can revolutionize your workflows and drive your business success.