Understanding the Importance of Training Data for Self-Driving Cars
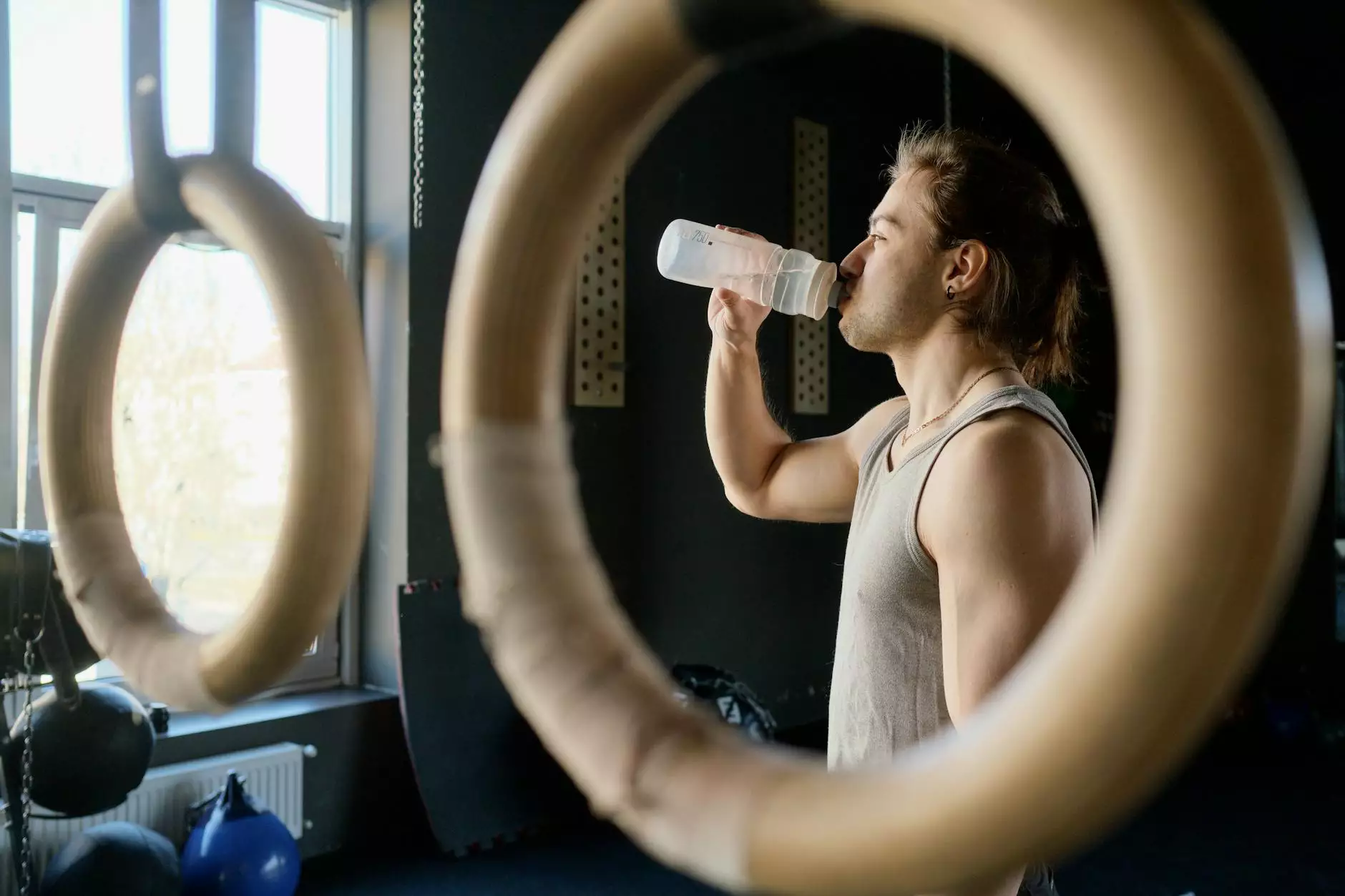
The Essential Role of Training Data in Autonomous Vehicles
In the rapidly evolving landscape of automotive technology, the development of self-driving cars stands out as one of the most exciting and transformative breakthroughs. However, at the very core of this innovation lies an element that is often underestimated: training data. This data serves as the foundational building block that allows autonomous vehicles to navigate and operate safely and efficiently in our dynamic world.
What is Training Data?
Training data refers to the vast quantities of information that machine learning models use to learn how to perform specific tasks. In the context of self-driving cars, this involves collecting data from various sources, including:
- Vehicle Sensors: Cameras, LIDAR, radar, and ultrasonic sensors collect real-time data about the vehicle's surroundings.
- Geospatial Data: Maps and geographic information systems (GIS) provide essential context about the environment.
- Driving Behaviors: Recorded data from human drivers, including decision-making processes in various scenarios.
This accumulated data is critical for training and refining algorithms that guide the vehicles' decision-making processes.
Types of Training Data for Self-Driving Cars
The training data is not just a single dataset; it comprises various types that fulfill different objectives. Understanding these types ensures a comprehensive approach to training autonomous vehicle systems. The primary types include:
1. Sensor Data
Sensor data includes inputs from cameras, LIDAR, and radar systems. These sensors gather real-time information about objects, distances, and environmental conditions, which are crucial for situational awareness.
2. Annotated Datasets
Annotated datasets are essential for supervised learning where data is labeled to indicate what the algorithm should recognize. For instance, identifying road signs, pedestrians, and other vehicles is vital for safe navigation.
3. Simulation Data
Using virtual environments to simulate various driving scenarios allows developers to test how self-driving algorithms respond to situations that may be rare in real life, ensuring thorough testing and safety.
4. Historical Traffic Data
Historical traffic data provides insights into patterns and behaviors in specific environments, aiding in predictive modeling and improving decision-making systems.
The Significance of Quality Training Data
The effectiveness of any autonomous vehicle system is immensely dependent on the quality of its training data. High-quality data leads to better model accuracy, which is fundamental for ensuring safety and operational reliability. This quality manifests in several ways:
- Completeness: A comprehensive dataset covers a diverse range of scenarios, including various weather conditions, times of day, and urban versus rural settings.
- Relevance: Data must accurately represent real-world conditions that the vehicle will encounter.
- Timeliness: The data collected should be recent enough to reflect current traffic patterns and rules, as these can evolve over time.
Challenges in Collecting Training Data
Despite its importance, gathering training data for self-driving cars comes with significant challenges, including:
- Cost: The technological infrastructure required to collect and process data can be exorbitantly expensive.
- Privacy Concerns: Collecting data, particularly from human drivers, raises privacy issues that must be rigorously managed.
- Data Volume: The sheer volume of data generated from sensors can be overwhelming, necessitating sophisticated handling and storage solutions.
- Bias in Datasets: If the data collected does not represent diverse driving conditions or populations, it may lead to biased algorithms that perform poorly in real-world applications.
Leveraging Advanced Technologies for Data Collection
To overcome challenges, the field of autonomous driving is leveraging various advanced technologies. These include:
- Cloud Computing: Cloud platforms enable efficient storage and processing of large datasets, making data accessibility easier for developers.
- AI-Driven Analytics: Artificial Intelligence (AI) can help in analyzing data for patterns that are critical in developing predictive models.
- Collaborative Data Sharing: Collaboration among companies can enhance the richness of data, pooling resources to create more complete datasets.
The Future of A.I. and Self-Driving Cars
As technology continues to evolve, so too will the methods employed in collecting and utilizing training data for self-driving cars. It's clear that AI will play an increasingly central role. Future advancements will likely include:
- Enhanced Visual Recognition: More advanced algorithms will improve the car's ability to recognize and respond to complex environments.
- Dynamic Data Updating: Vehicles may begin to update their training data in real-time from the cloud, refining their models continuously as they encounter new conditions.
- Interconnected Vehicles: The concept of V2X (Vehicle to Everything) communication will further expand the data pool, allowing vehicles to share insights about road conditions and safety hazards.
Conclusion
The journey towards fully autonomous driving is undeniably complex, with training data for self-driving cars serving as its lifeblood. Enhancements in data collection, algorithm training, and real-time adaptations will determine the success of these innovations. As stakeholders in the automotive industry work collaboratively to improve the efficacy and safety of self-driving cars, we stand on the brink of a transportation revolution that promises to change the way we travel. Understanding the significance of quality training data is not just beneficial; it is essential for paving the way for safer roads in the future.
This article is brought to you by Keymakr, your trusted source for home services, locksmith solutions, and cutting-edge technology insights.
training data for self driving cars